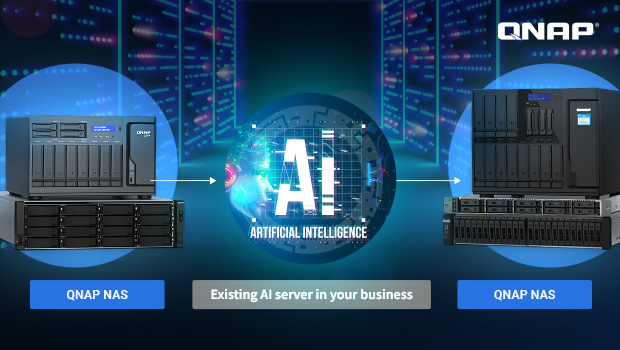
In the current buzz around artificial intelligence, while everyone’s attention is focused on computing power, which is considered as the “shovel for mining the AI gold mine”, the “warehouse” used to store the gold mine and processed products is actually also indispensable and far more important than you might think.
The rapid development of generative AI has caused explosive growth of AI applications. While continually attracting attention and sparking people’s imagination, this technology has also brought revolutionary changes to various industries. In today’s fiercely competitive market environment, companies are constantly seeking ways to improve efficiency and competitiveness. The development of artificial intelligence has brought tremendous opportunities for businesses, especially in the area of generative AI systems. These AI-powered systems can automate many tasks that previously required manual intervention, such as improving customer experience with self-service virtual customer service agents, enhancing contact center operations, significantly boosting employee productivity and creativity, expanding and accelerating marketing content creation, generating powerful sales content, brainstorming and developing new products, or automatically executing document data extraction and analysis.
However, to achieve these goals, enterprises still need a reliable and efficient storage architecture to support the training and deployment of AI models. For this purpose, QNAP NAS offers optimized enterprise AI development solutions.
On-premises deployment of AI model training ensures foolproof security and reliability
More and more enterprises choose to train AI models locally instead of relying on cloud services. Reasons for this include data security and privacy, cost control, performance and customization, as well as compliance requirements. When processing sensitive data, such as production records and financial data, on-premises training can ensure that the data is fully controlled by the enterprise for security and privacy protection. Certain industries and regions have stringent data storage regulations. On-premises training can help comply with these legal requirements and avoid compliance risks.
In addition, cloud computing is expensive, especially for large-scale and long-term training. By acquiring and maintaining their own infrastructure, enterprises can more effectively control long-term costs. Deploying dedicated hardware on-premises can avoid issues related to cloud resource sharing and network latency. Enterprises can also customize the software environment according to their own needs to ensure high performance and stability.
Role of data management and storage in AI development
According to IDC’s forecast, AI will generate 394ZB of data by 2028, implying a compound annual growth rate of 24% from 2023 to 2028. These forecasts demonstrate the growing demand for efficient and scalable AI storage solutions.
With the rapid development of AI technology, the creation and consumption of data are also increasing dramatically. The explosive growth of data is driving significant advancements in AI. The more data is created, the better the AI results, which leads to more data being stored in AI models. Nowadays, most AI is used to generate text, videos, images, and many other interesting content. All of this means that storage will become increasingly important in the future growth and evolution of AI.
Although AI is transforming lives and inspiring new applications, its core remains the utilization and generation of data. While processing and analyzing existing data, AI systems create new data that is often stored due to its practical value. At the same time, this data makes existing databases and additional data sources more valuable for model context and training, thus creating a self-reinforcing cycle. Increased data generation drives expansion of data storage, which in turn drives even more data generation.
In summary, the AI data cycle can be divided into six stages:
- Raw data archiving and content storage
This is the first step in the data cycle, securely and efficiently collecting and storing raw data from various sources. The quality and diversity of the data collected is crucial and sets the stage for everything that follows.
- Data preparation and import
In this stage, data is processed, cleaned, and transformed to prepare it for model training. Data center owners are implementing upgraded storage infrastructure, such as faster “data lakes” to cope with data preparation and import.
- AI Model training
At this stage, AI model undergoes iterative training to make accurate predictions based on the training data. Specifically, models are trained on high-performance supercomputers, which require specialized and high-performance storage equipment to run efficiently.
- Interfaceintegration and prompting
This stage involves creating user-friendly interfaces for AI models, including APIs, dashboards, and tools that combine context-specific data with end-user prompts. AI models will be integrated into existing Internet and client applications, enhancing them rather than replacing existing systems. This means maintaining existing systems while adding new AI computing requirements, thereby driving further storage demands.
- AI inference engine
The fifth stage is where the “AI magic” happens in real time. This stage involves deploying the trained models into a production environment, where they can analyze new data and provide on-the-fly predictions or generate new content. The efficiency of the inference engine is critical for timely and accurate AI response times, and requires comprehensive data analysis and excellent storage performance.
- Generate new content
The final stage is the creation of new content. Insights generated by AI models often produce new data, which is stored due to its value or relevance. While this stage closes the cycle, it also feeds back into the data cycle, driving continuous improvement and innovation by increasing the value of the data for future model training or analysis.
AI is not just about computing power and storage, it involves data management as well
The success of generative AI systems relies on high-quality data management and storage. For example, the Retrieval-Augmented Generation (RAG) architecture, which aims to “make large language models smarter,” relies on large databases to retrieve relevant information and generate meaningful responses. If data quality is poor or contains errors, it will directly affect the accuracy of retrieval results and the reliability of generated content.
Good data management can ensure data cleaning, orderly preprocessing, and improves data quality, which in turn enhances the performance of RAG models. Inaccurate data can cause user to lose confidence in the system, thus reducing its usage and acceptance. A vector database is a multi-dimensional vector data set. The increase in data quantity and dimensions will significantly increase storage space requirements. Insufficient storage space can lead to data write failures, resulting in data inconsistencies that affect the accuracy of retrieval results.
Internal data in the RAG architecture needs to maintain consistency and integrity across different storage nodes. Errors, inconsistencies or corruption in data backups may lead to inaccurate retrieval and generation of results. RAG models need to retrieve large amounts of data within a short time. A well-designed data storage structure and retrieval techniques can significantly improve retrieval speed, reduce latency, and enhance user experience. Inefficient data access will increase the computational burden on the system and reduce overall performance.
QNAP NAS can be entrusted with the crucial task of storing AI raw data
QNAP NAS offers various features to effectively support enterprises’ AI model training needs. QNAP NAS can accommodate large volumes of raw data (including videos and photos) and supports multiple storage protocols to achieve seamless access locally and in the cloud. This makes it well-suited for storing raw data from various platforms. QNAP NAS has high scalability, efficient data transmission, flexible protocol support, and powerful data protection capabilities. It provides PB-level storage capacity and has advanced snapshot and backup technology.
Using QuObjects to create S3 object storage on QNAP NAS, developers can easily migrate data stored in the cloud to the NAS. In a RAG architecture, vector databases are typically deployed and managed using Docker container. QNAP NAS supports not only container virtualization but also container import/export. This allows developers to back up and migrate multiple containers, and seamlessly access data across different platforms such as Windows, Linux, and macOS. The robust sharing capabilities significantly enhance data management efficiency for data cleaning personnel. Qsirch can be used to identify and remove duplicate, incomplete, and inaccurate data within datasets, enhance data quality and make it more suitable for training and using RAG models.
If issues or accidental deletions occur during the data cleaning process, developers can use Snapshot to restore the original data to a previous version, which can prevent data loss and save time. QNAP NAS supports various RAID configurations and also offers a range of built-in backup tools for developers to create backups of raw data.
QNAP NAS supports fine-grained permission settings. Specific access permissions can be set for each file and folder to ensure that only authorized users can access and modify data. WORM can prevent unauthorized data modification and ensure data integrity and consistency, which is particularly important for the data retrieval and generation process in a RAG architecture.
The ultra-high IOPS and low-latency features of All-flash NAS ensure that data can be retrieved and processed quickly. QNAP offers one of the most comprehensive all-flash NAS solutions in the industry, providing low latency and high performance to meet the frequent data access and processing needs in a RAG architecture. Additionally, 25/100GbE high-speed networking enables faster data transfer between devices, which is crucial for RAG architectures that require frequent read and write operations on large datasets. This significantly reduces data transfer latency and enhances overall system efficiency.
An efficient and cost-effective perfect solution
QNAP offers several NAS models suitable for raw data storage and RAG architecture storage/backup, including TDS-h2489FU, TS-h2490FU, TS-h1090FU, TS-h3087XU-RP, TS-h1677AXU-RP, TS-h1290FX, TS-h1277AFX, TVS-h1288X and TVS-h1688X. These models not only provide efficient and cost-effective solutions but also feature robust data protection and scalability to meet the needs of enterprises of all sizes. Whether used as raw data storage servers or RAG-structured storage/backup servers, these models can provide perfect solutions to help enterprises achieve an efficient, lean and highly scalable AI development environment.
By using QNAP NAS to support on-premises AI model training, enterprises can gain significant advantages in data security, cost control, performance optimization and compliance. The efficient storage solutions provided by QNAP can meet the various needs of modern enterprises in the AI development process, ensure high quality and high reliability of data, and enhance enterprises’ overall competitiveness.